Fachgebiet Verteilte Systeme und Betriebssysteme (DOS)
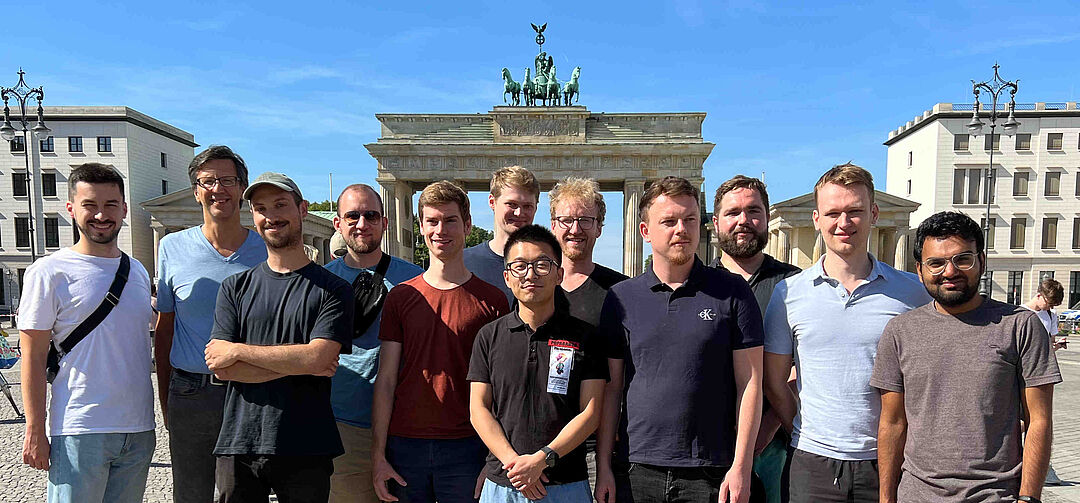
Kontakt
E-Mail:
service ∂ dos.tu-berlin.de
Tel:
+49 30 314-25154
Fax:
+49 30 314-21114
Adresse:
Verteilte Systeme und Betriebssysteme
Sekretariat TEL 12-5
Ernst-Reuter-Platz 7
10587 Berlin
Fachgebietsleitung | ||||||
👤 Kao, Odej | ✉️ odej.kao ∂ tu-berlin.de | 📞 +49 30 314-28970 | TEL 1206 | |||
Sekretariat | ||||||
👤 Bechstein, Jana | ✉️ jana.bechstein ∂ tu-berlin.de | 📞+49 30 314-25154 | TEL 1208 | |||
Systemadministration | ||||||
👤 Kitanova, Britta | ✉️ britta.kitanova ∂ tu-berlin.de | 📞 +49 30 314-78688 | TEL 1212 | |||
Wissenschaftliches Personal und Doktoranden | ||||||
👤 Acker, Alexander | ✉️ alexander.acker ∂ tu-berlin.de | 📞 +49 30 314-25397 | TEL 1204 | |||
👤 Aslan, Haci Ismail | ✉️ aslan ∂ tu-berlin.de | 📞 +49 30 314-2620 | TEL 1210 | |||
👤 Bader, Jonathan | ✉️ jonathan.bader ∂ tu-berlin.de | 📞 +49 30 314-19003786 | TEL 1211 | |||
👤 Becker, Sören | ✉️ soeren.becker ∂ tu-berlin.de | 📞 +49 30 314-78592 | TEL 1204 | |||
👤 Behnke, Ilja | ✉️ i.behnke ∂ tu-berlin.de | 📞 +49 30 314-73884 | TEL 1204 | |||
👤 Bogatinovski, Jasmin | ✉️ jasmin.bogatinovski ∂ tu-berlin.de | 📞 +49 30 314-26978 | TEL 1209 | |||
👤 Geldenhuys, Morgan | ✉️ morgan.geldenhuys ∂ tu-berlin.de | 📞 +49 30 314-73840 | TEL 1213 | |||
👤 Irion, Julius | ✉️ irion ∂ campus.tu-berlin.de | 📞 +49 30 314-19003521 | TEL 1217 | |||
👤 Kilian, Paul | ✉️ p.kilian ∂ tu-berlin.de | 📞 +49 30 314-19003521 | TEL 1217 | |||
👤 Nair, Sanika | ✉️ nair ∂ tu-berlin.de | 📞 +49 30 314-73470 | TEL 1214 | |||
👤 Nedelkoski, Sasho | ✉️ nedelkoski ∂ tu-berlin.de | 📞 +49 30 314-24813 | TEL 1203 | |||
👤 Paulus, Bela | ✉️ | 📞 +49 30 314-73470 | TEL 1214 | |||
👤 Scheinert, Dominik | ✉️ dominik.scheinert ∂ tu-berlin.de | 📞 +49 30 314-25286 | TEL 1205 | |||
👤 Steinke, Marvin | ✉️ marvin.steinke ∂ campus.tu-berlin.de | 📞 +49 30 314-73470 | TEL 1214 | |||
👤 Styp-Rekowski, Kevin Marcel | ✉️ styp-rekowski ∂ tu-berlin.de | 📞 +49 30 314-27494 | TEL 1210 | |||
👤 Treide, Nico | ✉️ nico.treide ∂ campus.tu-berlin.de | 📞 +49 30 314-73470 | TEL 1214 | |||
👤 Wiesner, Philipp | ✉️ wiesner ∂ tu-berlin.de | 📞 +49 30 314-19003941 | TEL 1218 | |||
👤 Wilhelm, Patrick | ✉️ patrick.wilhelm ∂ tu-berlin.de | 📞 +49 30 314-19003941 | TEL 1218 | |||
👤 Will, Jonathan | ✉️ will ∂ tu-berlin.de | 📞 +49 30 314-19003786 | TEL 1211 | |||
👤 Wittkopp, Thorsten Philipp | ✉️ t.wittkopp ∂ tu-berlin.de | 📞 +49 30 314-25286 | TEL 1205 | |||
👤 Xu, Jiawen | ✉️ jiawen.xu ∂ campus.tu-berlin.de | 📞 +49 30 314-26978 | TEL 1209 | |||
👤 Yu, Qiao | ✉️ qiao.yu ∂ campus.tu-berlin.de | 📞 +49 30 314-26978 | TEL 1209 | |||
Gastprofessor_innen | ||||||
👤 Madjarov, Gjorgji | ||||||
👤 Shui, Yu |